A couple of weeks ago, a new paper came out in JAMA Pediatrics with the title “Maternal Obesity and Risk of Sudden Unexpected Infant Death.” The central argument in the paper is that rates of sudden unexpected infant death (SUID) are higher for mothers who are classified as obese than those who are not.
This is a difficult topic to think, or write, about. First and foremost, it is the worst nightmare of parents to lose a child. And second, linking this risk to a particular individual characteristic smacks of judgment and blame. For data reasons, this research also focuses only on BMI, which we know isn’t a direct measure of health.
Despite the difficulty, this is important. There are many recommendations for safe sleep designed to prevent SUID, but we know they are not perfectly followed. I have long advocated for more thoughtful nuance in how we present these recommendations — for talking to people about gradations of risk.
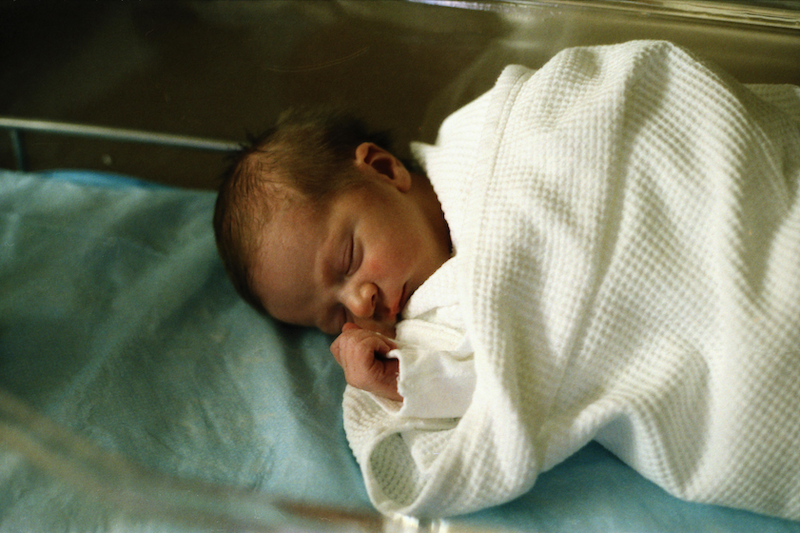
Part of this nuance is talking to people about their individual risk. We know, for example, that preterm babies are at increased risk of SUID. Parents of preterm babies should be counseled that safe sleep recommendations apply even more strongly to them. Similarly, if obesity is associated with a higher risk of SUID, this is crucial to communicate, even if it is hard to hear.
In contrast, if this link is not well supported in the data, that would also be helpful to understand for future conversations with your doctor about risks. This article is straightforward: I’m going to unpack the details of this paper and talk about what we learn and what we do not. (Spoiler alert: we don’t learn much and I share my bigger-picture thoughts at the end.)
Background and basic setup
The structure of this paper is fairly simple. The authors start with data from all births in the U.S. between 2015 and 2019. The CDC provides these data, which include information recorded on the birth certificate (maternal BMI, along with other risk factors). These data are also linked to infant deaths, including cause. The authors are therefore able to identify all SUID deaths in this group.
SUID is a category that is broader than sudden infant death syndrome (SIDS). SIDS deaths are intended to be defined as deaths where an infant was found (say, in their crib) having died. SUID includes these deaths, but also those classified as due to suffocation or to “ill-defined and unknown cause.” The inclusion of suffocation here is a key detail, since one of the possible mechanisms for obesity to impact infant death is through an increased risk of suffocation. The authors focus only on this broader definition, so we cannot directly comment on which precise cause of death is driving their results.
There are almost 19 million births in this sample, and 16,545 SUID deaths over the period.
The analysis in the paper compares SUID death rates across maternal pre-pregnancy obesity categories. The authors attempt to adjust for other differences across groups so they can isolate the impact of maternal obesity (more on this below).
What do the results show?
In a paper like this, the central results are presented as “odds ratios,” which is the increased risk of the outcome for a given group relative to some baseline reference group. In this case, the reference group is individuals with a BMI between 18 to 25 (what is referred to in medical terms as a “normal” BMI). So if we say the odds ratio for those with a higher BMI is 1.5, that means their risk is 50% higher than the “normal” BMI group.
These odds ratios can be a bit hard to absorb, because a 50% increase in risk means something different if the baseline risk is 1 in 10 versus if it is 1 in 1,000. What is more helpful, at least to me, is to use these odds ratios to produce predicted risk levels by group.
The graph below shows the estimated risk of SUID per 1,000 births, for mothers grouped by pre-pregnancy BMI. There are two sets of bars. The first one just captures the raw differences across the groups. The second set of bars adjusts for a set of controls — effectively, these are the differences holding constant maternal age, race, education, marital status, and smoking behavior, as well as paternal age and race, child sex, and birth order.
In the unadjusted analyses, you can see that the risks are higher for all groups who are not in the “normal weight” category. Once adjusted for other differences across mothers, however, the elevated risk appears only in groups with a pre-pregnancy BMI of above 30.
On its face, this suggests an elevated risk of SUID for mothers with obesity. It should be said that this elevated risk is fairly small, especially for those with a BMI under 40. Even at the top: the risk of SUID is elevated by 0.29 deaths per 1,000 births for women with a BMI over 40 relative to those with a normal BMI.
The core issue: causality versus correlation
A core issue in this paper, or any paper like it, is the question of whether the differences reflect correlation or causation. The concern is that although the authors do adjust for some differences across mothers, there may be other differences — other than maternal BMI — that drive the results. These other characteristics are things we cannot easily see or capture in data. This is what we sometimes call “unobservable confounding” or “residual confounding” or “omitted-variable bias.” It is a topic of my research and writing here.
To be thoughtful about this concern, we need to ask what the goal of the research is. In this case, the researchers’ goal (I asked them) was to estimate the total effect of maternal weight on SUID. They wanted to ask, if you were to advise someone pre-pregnancy about the risk of SUID for their infant, how would weight factor into that advice? Because this is their approach, the authors do not want to adjust their analyses for differences across infants at birth. In particular: we know that preterm birth is more likely for mothers with obesity. Prematurity is also linked with SUID. One mechanism for the overall relationship, then, is through prematurity. The authors want to capture this as part of the relationship they estimate, so in their main analyses, they do not adjust for prematurity.
This is internally consistent, but it strikes me as a bit odd from a practical public health standpoint. When we are giving advice to mothers about safe sleep, we are generally doing so after the baby has arrived. At that point, we know quite a lot more about the infant — including things like prematurity, birth weight, other complications — that contribute to risk. From the standpoint of advice-giving, I might have thought we’d want to estimate these effects holding constant characteristics of the infant. That was not the goal of the paper, though.
So the authors do not adjust for differences across the infant. However, there are differences between mothers that they do adjust for. The primary analyses in the paper adjust for variables like maternal education, age, race, and smoking. There are other variables they do not adjust for. For example, heavy alcohol consumption is a possible confound — again, known to be correlated with obesity and with SUID—that they do not adjust for.
A broader concern is that the measures the authors have of socioeconomic status — maternal education, whether the birth is privately or publicly insured — are imperfect. We know that families with fewer resources can struggle more with managing safe sleep environments. If the authors are only measuring these variables imperfectly, their results may still overstate the true relationship between weight and mortality.
If the authors were to take a richer set of variables into account, their results would almost certainly show smaller differences across groups. The question is whether the differences would literally become statistically insignificant. It is extremely difficult, if not impossible, to know. Because the variables we worry about are unobserved, we are stuck. Any arguments about whether the results are believably causal or not are just that: arguments.
In my own work, I’ve developed techniques to evaluate the scope of this problem based on the idea that we learn something about the importance of these unobserved variables from the importance of the observed ones. Without getting extremely technical: in the approaches I use, the fact that the effects here are much smaller once the authors take into account the controls (remember the unadjusted vs. adjusted data in the graph above?) gives a clue that they would probably be even smaller if the authors were able to take into account more variables.
The authors themselves take a different approach, relying on something called an E-value. Unfortunately, there is little to guide whether particular values of this measure are reassuring or not.
The bottom line
In an ideal world, research in public health would tell us something about what guidance we should give people (or about policy). I struggle to see how to fit the results of this paper into that goal. Even in concept, the results do not speak directly to the guidance that we would want to give people at birth, since after birth we would know much more about the infant. More generally, I am quite confident that including richer socioeconomic controls would make the results even smaller than they already are. At a certain point, the effect becomes so small that it’s not clear it is an important factor in our messaging.
Beyond this, I believe we should always have an added moment of caution in giving guidance. When we do not have clear or convincing data – as I’d argue is true here – people are often told inconsistent or untrue things. That’s not just about BMI, it happens all the time during pregnancy. People hear mixed messages, or they interpret that they are to blame if something bad happens, or both. This isn’t good for patients, and it isn’t good for the patient-provider relationship.
In the end, it isn’t that we should hide data from people – I’m the last person who would suggest that. But we should strive for better data here, and in many areas of pregnancy.
Community Guidelines
Log in
My (slightly rhetorical) question here is: what is the *action* these authors suggest should be taken based on the outcome of the study? They suggest that this is intended to give guidance to mothers before the baby is born. The implication, to me, is that the guidance would be: “lose weight so you don’t kill your baby”. I’m probably assuming a LOT about the underlying intent here, but if that’s the guidance we would give, it is terrible. We know that a doctor telling a patient “lose weight” is ineffective in changing the weight of the patient, but it does make the patient not want to go to the doctor. I guess the bottom line is: I wish research that focused on BMI would try to answer the question: how can we actually support people in real life? If that were the intent, we’d take into account factors about the real life infant to help parents keep their baby safe during sleep.
https://highline.huffingtonpost.com/articles/en/everything-you-know-about-obesity-is-wrong/
Question: the first chart shows being underweight also has elevated suid risk, but it is flat to normal weight in the second chart. Can Emily or another smarty pants here explain why that would be? It seems dubious to me, but I’d like the details before I sound off 😉
I would guess that being underweight is highly correlated with the control factors (e.g., smoking and socioeconomic status).