I read about a study saying caffeine in pregnancy makes children shorter. True?
—Anonymous
Many of you read about this! (Thanks, CNN, for the typical scary headline). The study is here, if you missed it. Broad strokes: The authors use two cohort studies that record information on caffeine during pregnancy and child height and weight. In both, they document a link between caffeine concentration measured in maternal blood and child height. Of note is that one of the studies uses relatively recent data and measures children at only one age; the other uses older data from the 1960s but includes measurements at many ages.
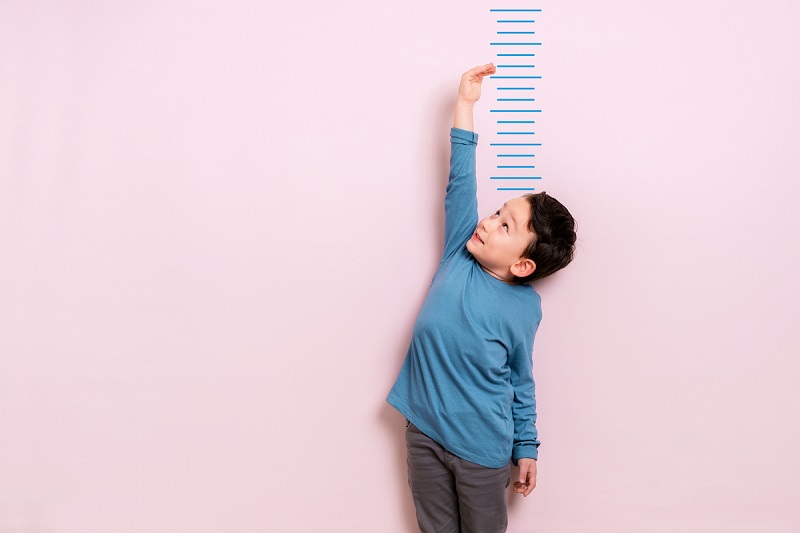
The issue with this study is similar to all studies of this type. It’s observational, not randomized, and there are very significant differences between the groups who drink coffee and those who do not. Important ones include large differences in racial composition, differences in number of children, and differences in socioeconomic status. These appear in both the more recent data and the older data.
The most compelling results in the paper appear using the older data (in the figures and supplemental appendix), where the authors show that as children age, differences appear in height between those with higher and lower caffeine exposure during pregnancy. These differences in their data max out at about 2 centimeters, which isn’t obviously clinically significant, but it is statistically significant.
I am skeptical, as always, about studies that rely on this type of observational data. The controls are nearly always imperfect; in this case, for example, they do not have any information on paternal height, which is a likely confound. But here I actually have a more specific idea of what might be driving the results, rather than just general complaining.
The primary analysis in the older data set involves estimating the relationship between height and caffeine consumption, interacted with age. In other words, the author look to see whether as kids age, those who were exposed to more caffeine become relatively shorter. When you do this analysis and you include controls (for things like race or socioeconomic status), you also need to interact those controls with age. If, for example, there are different growth trajectories by race or income (which is very plausible), then the bias will not be fixed by just controlling for these variables overall.
This is admittedly a bit in the weeds (I’m sorry! It’s my job!), but I suspect it is what is driving these results. It’s a further illustration of the issues with non-randomized studies and how you have to be really, really careful in interpreting results.
Community Guidelines
Log in